第32回研究会
開催日:
2018年10月3日 (水) 18:00-19:00
会場:
丸の内永楽ビルディング18階 首都大学東京 丸の内サテライトキャンパス
報告者:
Prof. Fumiya Akashi (Waseda University, Research Institute for Science and Engineering)
タイトル:
Robust statistical inference for nonstandard time series models and related topics
概要Abstract
This talk develops robust statistical inference procedures for various nonstandard time series models. In the context of time series analysis, statistical treatment of heavy tails and long-range dependence has attracted much attention. The problems brought by such nonstandard aspects often appear. For example, the rate of convergence of fundamental statistics contains unknown tail/Hurst index, and the limit distributions of classical statistics become intractable. As a result, it is infeasible to determine cut-off points for confidence intervals or critical values for test statistics in practice. To overcome such hurdles, we employee the least absolute deviations regression, self-normalization and self-weighting methods, and construct the robust inference procedures in the following three topics:
(1) The first part of this talk considers robust interval estimation for time series regression models whose innovation and covariate processes have possibly heavy-tails and/or long-range dependence. To make inference for such model, we introduce the self-normalized subsampling method following Akashi et al. (J. Time Ser. Anal. 39:417-432, 2018). As a result, we can construct confidence region for the regression parameter of the model without knowledge of tail/Hurst-indices of the underlying process.
(2) The second part of this talk introduces the self-weighted generalized empirical likelihood (GEL) statistic proposed by Akashi (Stat. Inference Stoch. Process 20(3):291-313, 2017) and its application to a change point detection problem (Akashi et al. (J. Time Ser. Anal. DOI: 10.1111/jtsa.12405, 2018)). The self-weighting method introduced by Ling (2005) enables us to construct a robust test statistic whose limit distribution is pivotal, regardless of whether the model has finite variance of not. We extend Ling (2005)’s method to the GEL statistics and elucidate the limit distribution of the proposed statistic. Some simulation experiments illustrate the desirable properties of the proposed method in practical situations.
(3) The third part of this talk provides a robust inference procedure in directional data analysis. We first review the recent development in directional statistics, and second introduce new robust inference method for circular-linear regression models. Since the extension of the methodologies in the “linear” statistics to the “circular” statistics is not trivial, the proposed method includes a lot of novel aspects.
お申込み方法Registration
第32回研究会へ参加される方は必ず事前登録をお願いいたします。 参加登録の締切は10月2日(火)です。
下記のいずれかの方法で「お名前・所属機関・電子メールアドレス」をご連絡ください。
※参加費は無料です。
WEBからの登録
本ページにあります「REGISTER HERE」ボタンをクリックいただき、お申し込みフォームよりご登録ください。
電子メールでの登録
finance●tmu.ac.jpまで「お名前・所属機関・電子メールアドレス」をご連絡ください。
(※ご連絡時は●を@に置き換えてご使用下さい。)
当日のご案内Information
当日は丸の内永楽ビルディング3階の受付にて、首都大学東京のセミナーへ 参加する旨とご所属、お名前を申し出てください。
ビジターカードが発行されますので、18階の首都大学東京丸の内サテライト キャンパスまでお越しください。
金融工学研究センター概要
Research Center for Quantitative Finance
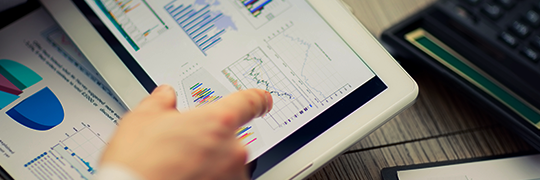
イベント情報
Event
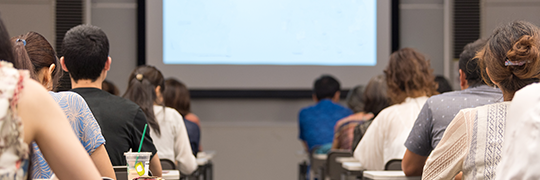