第95回研究会
開催日:
2025年1月10日(金) 15:20-17:30
会場:
丸の内永楽ビルディング18階 東京都立大学 丸の内サテライトキャンパス
(Zoomでも同時配信します)
報告者:
Silvana Pesenti 氏(Assistant Professor, University of Toronto)
Sebastian Jaimungal 氏(Professor, University of Toronto)
タイトル:
Static and dynamic robust risk measures
Risk Budgeting Allocation for Dynamic Risk Measures
プログラムProgram
15:20-16:20 Silvana Pesenti 氏(Assistant Professor, University of Toronto)
「Static and dynamic robust risk measures」
This talk is focused on distributionally robust risk measures, which are the largest value a risk measure can attain within an uncertainty set. Uncertainty sets are often characterised by balls around a reference distribution, thus containing plausible alternative distributions. I first discuss worst-case distortion risk measures for Wasserstein uncertainty sets and alternatives uncertainty sets that arise from asymmetric optimal transport divergences. Second, I discuss the dynamic setting, where the risk of stochastic processes is evaluated using time-consistent dynamic risk measures. In the dynamic setting I introduce dynamic robust risk measures and dynamic uncertainty sets and study conditions on the uncertainty sets that lead to well-known properties of dynamic robust risk measures, such as convexity and coherence. We proof necessary and sufficient conditions for a robust dynamic risk measures to be time-consistent and to admit a recursive representation.
16:30-17:30 Sebastian Jaimungal 氏(Professor, University of Toronto)
「Risk Budgeting Allocation for Dynamic Risk Measures」
We define and develop an approach for risk budgeting allocation — a risk diversification portfolio strategy — where risk is measured using a dynamic time-consistent risk measure. For this, we introduce a notion of dynamic risk contributions that generalise the classical Euler contributions and which allow us to obtain dynamic risk contributions in a recursive manner. We prove that, for the class of dynamic coherent distortion risk measures, the risk allocation problem may be recast as a sequence of strictly convex optimisation problems. Moreover, we show that any self-financing dynamic risk budgeting strategy with initial wealth of 1 is a scaled version of the unique solution of the sequence of convex optimisation problems. Furthermore, we develop an actor-critic approach, leveraging the elicitability of dynamic risk measures, to solve for risk budgeting strategies using deep learning.
[ joint work with Silvana M. Pesenti, Yuri F. Saporito, and Rodrigo S. Targino]
講師ご略歴Profile for speakers
Silvana Pesenti is an Assistant Professor in Insurance Risk Management at the Department of Statistical Sciences at the University of Toronto. Silvana was named the 2022 Rising Star in Quant Finance by Risk.net for the paper Portfolio Optimisation within a Wasserstein Ball. She received the 2020 Peter Clark Best Paper Prize for her research paper “Reverse Sensitivity Testing: what does it take to break the model?” from the Institute and Faculty of Actuaries (IFoA). In 2019, Silvana was awarded the Dorothy Shoichet Women Faculty Science Award of Excellence. Silvana Pesenti is an Associate Editor of Computational and Applied Mathematics (since 2022) an Associate Editor of Annals of Actuarial Science (since 2023), and on the Editorial Board of Applied Mathematical Finance (since 2023) and of ASTIN Bulletin (since 2023).
Dr. Sebastian Jaimungal is a Full Professor of Mathematical Finance at the University of Toronto and is currently the Chair of the Department of Statistical Sciences. He is a Fellow of the Fields Institute for Mathematical Sciences, an Associate Member of University of Oxford, Oxford-Man Institute for Quantitative Finance, a manager editor at Quantitative Finance, associate editor at SIAM J. Financial Mathematics (among other journals), and a former chair of the SIAM activity group on Financial Mathematics. He is a co-author of graduate textbook “Algorithmic and High-Frequency Trading” published by Cambridge University Press, and his research interests span stochastic control, mean field games, and reinforcement learning.
お申込み方法Registration
第95回研究会へ参加される方は必ず事前登録をお願いいたします。
参加登録の締切は1月8日(水)です。
下記のいずれかの方法で「お名前・所属機関・電子メールアドレス」をご連絡ください。
開催日当日の午前までにご参加のために必要なURLを記載したメールをお送りします。
※参加費は無料です。
WEBからの登録
本ページにあります「REGISTER HERE」ボタンをクリックいただき、お申し込みフォームよりご登録ください。
電子メールでの登録
finance●tmu.ac.jpまで「お名前・所属機関・電子メールアドレス」をご連絡ください。
(※ご連絡時は●を@に置き換えてご使用下さい。)
ご参加情報は、東京都立大学金融工学研究センター、講演者により共有され、当セミナーの円滑な運営のために利用します。
金融工学研究センター概要
Research Center for Quantitative Finance
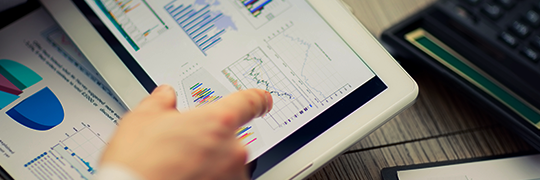
イベント情報
Event
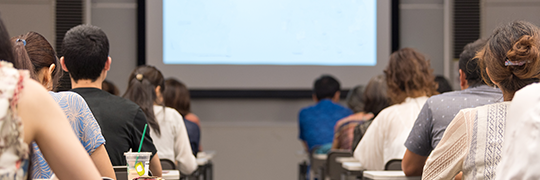